A global collaborative analysis group has developed a picture recognition expertise that may precisely decide the basic composition and the variety of cost and discharge cycles of a battery by analyzing solely its floor morphology utilizing AI studying.
Professor Seungbum Hong from the Korea Superior Institute of Science and Know-how (KAIST) Division of Supplies Science and Engineering, in collaboration with the Electronics and Telecommunications Analysis Institute (ETRI) and Drexel College in the US, has developed a way to foretell the most important elemental composition and charge-discharge state of NCM cathode supplies with 99.6% accuracy utilizing convolutional neural networks (CNN).
The paper is printed within the journal npj Computational Supplies.
The analysis group famous that whereas scanning electron microscopy (SEM) is utilized in semiconductor manufacturing to examine wafer defects, it’s hardly ever utilized in battery inspections. SEM is used for batteries to research the scale of particles solely at analysis websites, and reliability is predicted from the damaged particles and the form of the breakage within the case of deteriorated battery supplies.
The analysis group determined that it could be groundbreaking if an automatic SEM could possibly be used within the strategy of battery manufacturing, identical to in semiconductor manufacturing, to examine the floor of the cathode materials to find out whether or not it was synthesized in line with the specified composition and that the lifespan could be dependable, thereby decreasing the defect charge.
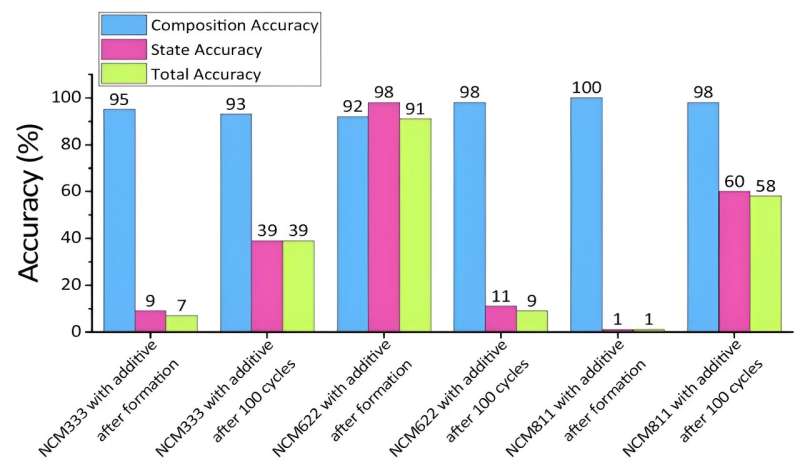
The researchers skilled a CNN-based AI relevant to autonomous automobiles to be taught the floor photos of battery supplies, enabling it to foretell the most important elemental composition and charge-discharge cycle states of the cathode supplies. They discovered that whereas the tactic might precisely predict the composition of supplies with components, it had decrease accuracy for predicting charge-discharge states.
The group plans to additional prepare the AI with numerous battery materials morphologies produced by totally different processes and in the end use it for inspecting the compositional uniformity and predicting the lifespan of next-generation batteries.
Professor Joshua C. Agar, one of many collaborating researchers of the venture from the Division of Mechanical Engineering and Mechanics of Drexel College, stated, “In the future, artificial intelligence is expected to be applied not only to battery materials but also to various dynamic processes in functional materials synthesis, clean energy generation in fusion, and understanding foundations of particles and the universe.”
Professor Seungbum Hong from KAIST, who led the analysis, acknowledged, “This analysis is critical as it’s the first on the planet to develop an AI-based methodology that may shortly and precisely predict the most important elemental composition and the state of the battery from the structural knowledge of micron-scale SEM photos.
“The methodology developed in this study for identifying the composition and state of battery materials based on microscopic images is expected to play a crucial role in improving the performance and quality of battery materials in the future.”
This analysis was performed by KAIST’s Supplies Science and Engineering Division graduates Dr. Jimin Oh and Dr. Jiwon Yeom, the co-first authors, in collaboration with Professor Josh Agar and Dr. Kwang Man Kim from ETRI.
Extra info:
Jimin Oh et al, Composition and state prediction of lithium-ion cathode by way of convolutional neural community skilled on scanning electron microscopy photos, npj Computational Supplies (2024). DOI: 10.1038/s41524-024-01279-6
The Korea Superior Institute of Science and Know-how (KAIST)
Quotation:
Examine employs image-recognition AI to find out battery composition and circumstances (2024, July 2)
retrieved 3 July 2024
from https://techxplore.com/information/2024-07-employs-image-recognition-ai-battery.html
This doc is topic to copyright. Aside from any honest dealing for the aim of personal research or analysis, no
half could also be reproduced with out the written permission. The content material is offered for info functions solely.